Intelligent Automation in Banking and Financial Services
Intelligent Automation in Banking and Financial Services
Intelligent Automation in Banking and Financial Services
Intelligent Automation in Banking and Financial Services
Anant Sharma
Anant Sharma
Anant Sharma
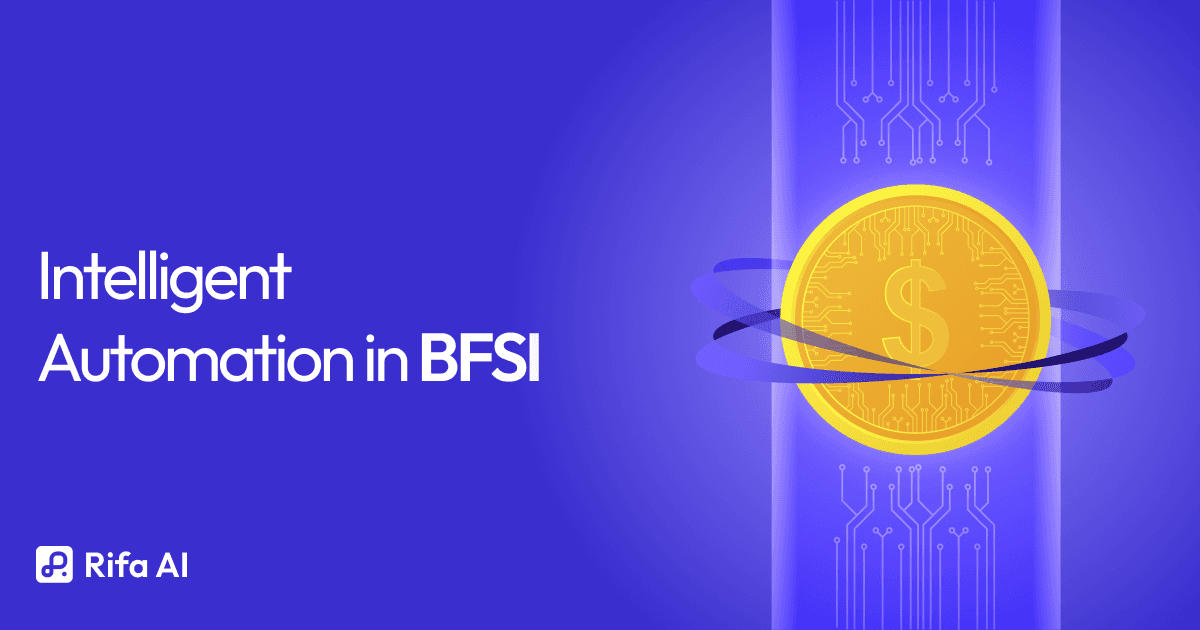
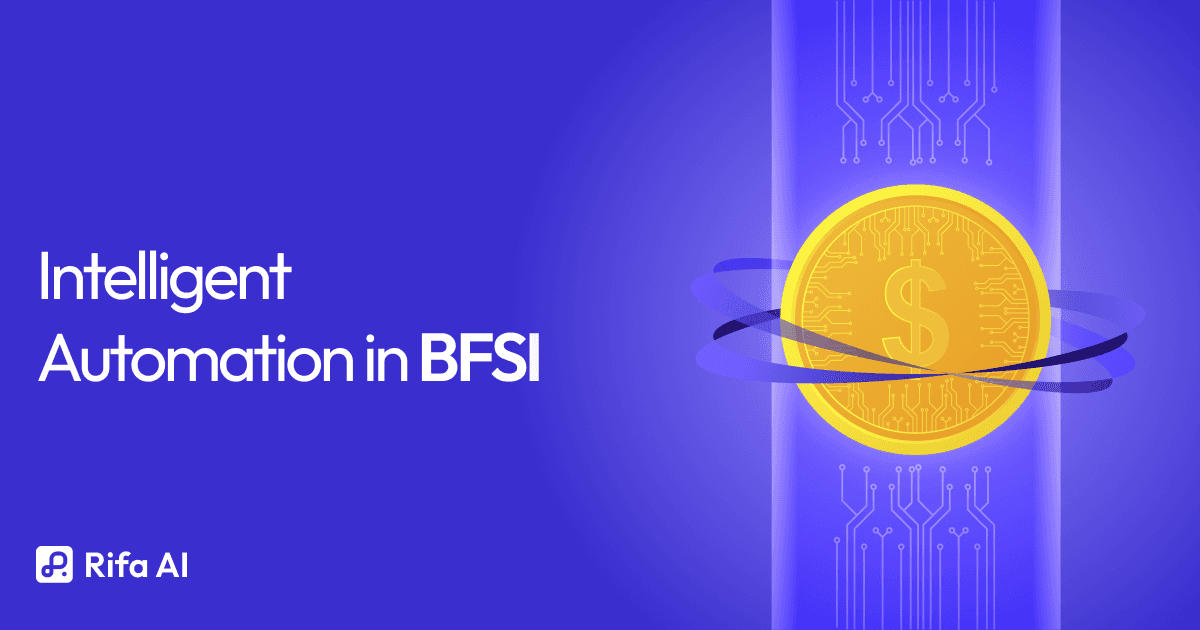
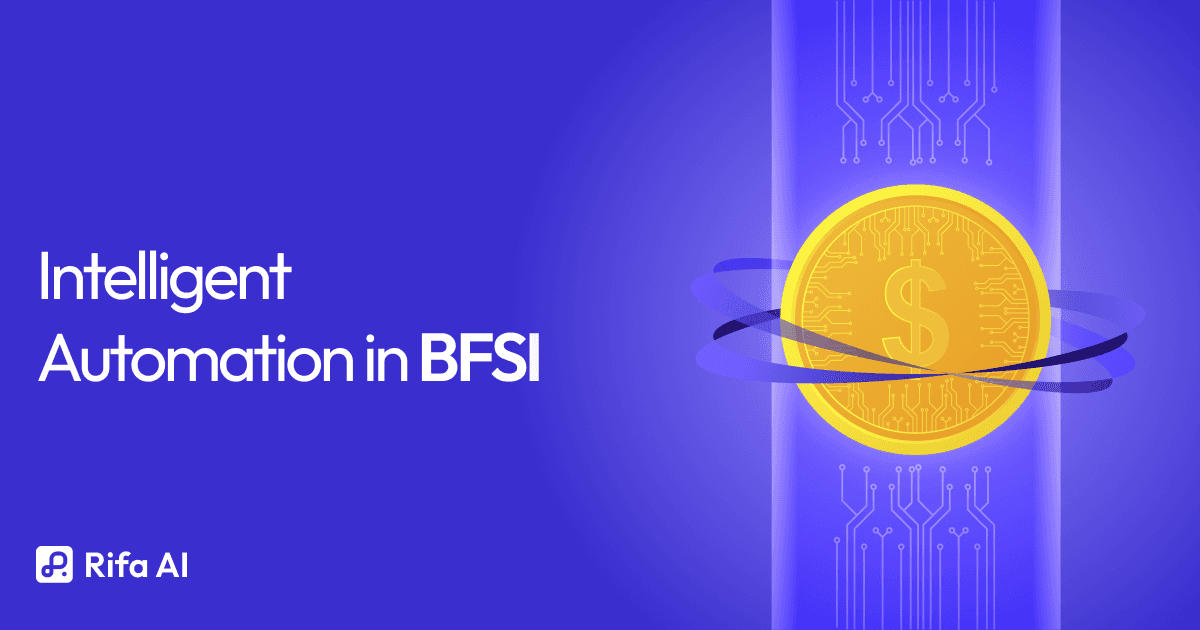
Introduction
The banking and financial services industry is under immense pressure to adapt to changing customer expectations, competitive pressures, and regulatory requirements. In this context, intelligent automation (IA) has emerged as a transformative solution.
IA combines robotic process automation (RPA) with advanced technologies like artificial intelligence (AI), machine learning (ML), and natural language processing (NLP). It enables systems to automate repetitive tasks and learn, adapt, and make decisions. According to Deloitte, 25% of enterprises using GenAI are forecasted to deploy AI Agents by 2025, growing to 50% by 2027. This trend is driven by the potential for IA to boost efficiency, reduce operational costs, and improve customer experiences.
Intelligent automation in finance is not just a technological upgrade but a strategic necessity for banks and financial institutions aiming to stay competitive.
Let’s start by defining the fundamental elements of IA and discussing how the banking and financial services sectors are changing as a result of its integration.
What is Intelligent Automation in Finance and Banking?
Intelligent automation incorporates various technologies in order to replicate human intelligence in task execution. RPA is primarily focused on automating repetitive tasks, but when combined with AI technologies, it can perform more complex tasks such as sentiment analysis, fraud detection, and predictive analytics.
An excellent example is Capital One’s Eno, an assistant who handles thousands of inquiries while learning from client interactions to offer personalized recommendations.
Rifa AI improves operational efficiency by integrating AI-driven tools that not only speed up debt recovery but also ensure compliance while saving both money and time. If you're automating billing and payment reminders, expediting dispute resolution, or improving customer service interactions, Rifa AI can help you optimize workflows and increase 40% recovery rates.
By using these technologies, banks often achieve more personalized customer experiences and faster financial analytics. After discussing how IA can change business outcomes, let’s examine how these technologies are applied in particular.
Applications and Benefits of Intelligent Automation in Finance
With its many uses and advantages, intelligent automation in finance can have an impact in the following ways:
AI-Led Chatbots to Improve Customer Service
AI-powered chatbots can provide personalized customer support. These bots use NLP to answer consumer questions, assist with financial products, and offer real-time support.
For example, HDFC Bank's Eva chatbot analyzes a customer’s past interactions, like checking credit card rewards or inquiring about loans. If a customer regularly asks about travel-related benefits, Eva might suggest a credit card with better travel perks or a loan with a lower interest rate. This upselling feels seamless and personalized, offering the right service at the right time, based on the customer’s behavior.
Streamlining Back-Office Operations
Automating manual and repetitive back-office processes like procurement, accounts payable, and loan origination saves time and reduces error. RPA tools are commonly used for data extraction, document verification, and compliance checks.
For example, the combination of RPA and Optical Character Recognition (OCR) technology accelerates loan application processing by automatically extracting and verifying data from submitted documents such as tax forms, identification documents, and income proofs. Typically, the OCR system scans and digitizes the documents uploaded by customers, while RPA workflows cross-check this digitized data against internal databases. If discrepancies are identified, such as mismatched income information, the RPA system promptly triggers an alert for further investigation.
Operational Efficiency and Customer Experience
Repetitive tasks can be automated by integrating RPA with AI. While RPA manages structured processes, AI elevates them with features like ML and NLP, enabling the processing of unstructured data such as fraud detection or customer inquiries. Predictive models enable proactive engagement, such as offering personalized financial advice or detecting potential fraud risks early.
For instance, JP Morgan has substantially reduced processing time and operational expenses by using AI to analyze contracts. The technology automatically reviews and scans contracts, extracting relevant details such as payment terms, renewal dates, and highlighting key clauses. By using AI to quickly process large volumes of contracts, JP Morgan has significantly reduced the time spent on manual reviews and minimized human error. The AI system also flags any discrepancies or potential risks, which are then escalated for further examination by human specialists.
Adopting IA helps you stay ahead of the competition by improving customer engagement and streamlining internal processes, which ensures greater dependability and efficiency.
Rifa AI is transforming the process of debt collection by using AI technology to processes and reduce operational costs. By incorporating AI-powered negotiation tools and automating key functions, Rifa AI has proven to improve collection efficiency, shorten recovery cycles, and improve overall payment conversion rates.
Reduce Operational Costs by Up to 70%: Streamline processes to enhance efficiency.
99% Accuracy: AI systems ensure precision in every interaction, minimizing errors.
Quick Deployment: Implement and go live in days without the need for developer or API support.
Agent Time Savings: Automate repetitive tasks, allowing your team to focus on more strategic projects.
2x Improved Payment Conversions: Boost customer engagement and improve payment success rates.
40% Faster Debt Recovery: Accelerate collection cycles and increase cash flow with AI-driven negotiation tools.
Rifa AI runs continuously, ensuring regulatory compliance while seamlessly integrating with your current systems. This transforms your collections and financial operations into more streamlined, customer-centric, and profitable processes.
As we move from technological integration to real-world use cases, it’s critical to acknowledge that implementing IA can present difficulties.
Intelligent Automation Uses
AI, ML, and RPA are among the technologies transforming core banking and financial services operations through intelligent automation in finance. Here are three important use cases:
Automating Regulatory and Compliance Reporting
Manual compliance procedures can be costly, time-consuming, and error-prone. By automating risk assessments, transaction monitoring, and report generation, IA streamlines these processes. Rifa AI fits perfectly into the automation of regulatory and compliance reporting by automating tasks such as transaction monitoring, data extraction, and report generation, helping you maintain up-to-date compliance without the manual effort.
It also simplifies real-time compliance monitoring, ensuring that ever-changing regulations are followed while minimizing human error. By improving accuracy and reducing costs, this automation allows institutions to focus on strategic initiatives and meet regulatory requirements.
Executive Dashboards for Management Reporting
Real-time executive dashboards powered by IA combine data from multiple sources and visualize it to enable prompt decision-making. These dashboards allow executives to monitor operational risks, market trends, and performance metrics. This immediate access to insights optimizes organizational strategies.
During market volatility, a European bank improved the efficiency of C-suite executives' decision-making by using IA to build dashboards that integrated real-time financial metrics.
Improving Data Structuring for Mergers and Acquisitions (M&A)
Analyzing large datasets to evaluate risks and synergy opportunities is a common step in M&A activities. IA organizes and processes unstructured data identifies trends and provides valuable insights. It supports risk assessments, predictive analytics, and integration planning, as well as valuation and due diligence.
A multinational bank used AI-driven automation to analyze operational data, regulatory requirements, and financial records during a recent M&A. This approach provided deeper insights into potential synergies and reduced analysis time by 40%.
By implementing IA, you can boost productivity, reduce costs, and maintain a competitive edge in the financial market.
By using AI-driven negotiation tools to speed up debt recovery and reduce operating expenses by up to 70%, Rifa AI integrates into your current systems. Through the automation of functions such as data extraction, communication management, and payment tracking, Rifa AI helps you maintain compliance. Find out how Rifa AI can optimize your workflows and deliver immediate, cost-effective outcomes.
Addressing key issues like choosing the best technology partners and preparing your staff for upcoming changes will lead to better data management and operational process optimization. Let’s go through IA’s considerations and challenges.
Challenges and Considerations of Intelligent Automation in Finance
Although implementing intelligent automation in finance presents many opportunities, certain challenges should be carefully considered. Key elements of IT integration and cultural change include:
Selection of Vendors and Integration
In finance, IA is often difficult and resource-intensive to integrate with legacy IT systems. Modern automation tools, such as RPA and AI-driven platforms, may need to be supported by the legacy systems that financial institutions typically rely on. To address this:
Work Together Early with IT: A strong partnership with IT teams ensures seamless system lifecycle design and prioritization. To prevent implementation bottlenecks, align automation objectives with IT's overarching strategic roadmap.
Vendor Compatibility: Select solutions that lessen disruptions by enhancing current infrastructure. For example, banks like Barclays have used RPA to streamline back-office operations, resulting in cost savings and increased efficiency without having to redesign their systems completely.
Data Security and Quality: Automation tools require reliable, consistent data. Maintaining strong cybersecurity and data governance is essential to mitigate risks as automation scales. Rifa AI smmothens repetitive tasks, such as communication, reminders, and payment tracking. This automation ensures data accuracy while optimizing efficiency and reducing the risk of human error..
Employee Concerns
The introduction of IA in finance can bring about changes in workplace culture and concerns about job security. Employees may need help to transition into new roles that require more technical skills or fear of being replaced. To overcome these challenges:
Open Communication: Ensure that staff members understand the goals and benefits of automation. Rather than presenting automation as a job replacement, frame it as a tool for reducing repetitive tasks. For instance, organizations like the Australia and New Zealand Banking Group have used automation to free up staff for higher-value tasks.
Invest in Reskilling: Offer reskilling programs to help employees work effectively with automation tools. Promoting a positive attitude through redeployment into strategic roles that emphasize human judgment and creativity is important.
Leadership and Change Champions: To address employee concerns and support automation efforts, engage leaders and early adopters as "change champions." These advocates can help build trust in the transformation process and demonstrate the benefits of intelligent automation in finance.
Both IT integration and cultural adaptation require a phased approach to ensure the technology supports business objectives and gains employee buy-in. By learning from successful case studies and continuously improving procedures, your institution can steer these challenges while optimizing the benefits of automation.
Let’s get into optimizing resources and data integrity, which are the next logical steps in implementing intelligent automation in finance.
Data and Resource Optimization by Intelligent Automation
IA transforms data handling and resource allocation in finance, which uses technologies such as RPA, machine learning, and AI-powered analytics. By applying tools for data integration and validation, you can ensure the reliability of your datasets. This is necessary for compliance, and fraud detection.
Forecasts for the Intelligent Document Processing (IDP) Sector's Growth: The IDP sector, which promotes automated unstructured data extraction, classification, and analysis, is anticipated to grow rapidly. The increasing need for real-time processing in banking tasks such as regulatory reporting, loan approvals, and customer onboarding drives this trend.
Role of ML and NLP: Specifically, the market for IDP is projected to expand significantly due to advances in ML and NLP. These technologies help financial firms manage vast volumes of documents effectively, reduce compliance risks, and increase customer satisfaction.
Rifa AI uses advanced technologies to efficiently manage and process vast amounts of data and documents. By automating the document data extraction process, Rifa AI reduces the likelihood of human error, ensuring high levels of accuracy and compliance. This capability allows businesses to smoothen operations, mitigate compliance risks, and improve operational efficiency.
The ultimate objective of intelligent automation is to increase productivity and reduce operating expenses. Let’s discuss its impact on employees and cost-saving efficiency.
Impact on Employees and Cost Efficiency
Banks can use IA technology to cut expenses, simplify operations, and free up staff members from tedious work, allowing them to take on more strategic responsibilities.
Cutting Back on Working Hours and Strategic Focus: Intelligent automation in finance reduces the amount of manual labor needed for tasks like data entry, document verification, and compliance checks by automating repetitive processes. For example, Standard Chartered’s Trade AI Engine significantly reduces the need for you in routine tasks by using ML to analyze complex trade documents. Employee productivity increases as a result, with more time to concentrate on critical, thinking-intensive tasks like strategy development and customer engagement.
Cost Savings as a Result of Improved Workflows: Intelligent automation reduces costs by replacing inaccurate manual procedures with accurate and reliable automated workflows. This not only improves accuracy but also lowers operating expenses. Compliance and reduced operational risks benefit financial institutions. For example, Banco Santander’s Kairos AI tool promotes reducing the costs associated with risk mismanagement.
The successful adoption of IA by banks improves their operational resilience and positions them as leaders in the industry.
Conclusion
Intelligent automation (IA) in finance is improving customer experiences and operational efficiency. By combining technologies like robotic process automation (RPA), artificial intelligence (AI), and machine learning (ML).
By automating repetitive tasks, IA empowers employees to focus on higher-value activities such as strategic planning and customer engagement, promoting a culture of innovation. Leading banks have used automation platforms to improve data analytics, integrate legacy systems, and roll out new services quickly. Bank operations innovation and competition are all changed by the strategic implementation of intelligent automation in finance.
Rifa AI is in charge of the ongoing revolution of intelligent automation, transforming business collections. In addition to streamlining debt recovery, Rifa AI's AI-driven solutions reduce operating expenses, improve payment conversions, and ensure seamless integration with existing systems.
Introduction
The banking and financial services industry is under immense pressure to adapt to changing customer expectations, competitive pressures, and regulatory requirements. In this context, intelligent automation (IA) has emerged as a transformative solution.
IA combines robotic process automation (RPA) with advanced technologies like artificial intelligence (AI), machine learning (ML), and natural language processing (NLP). It enables systems to automate repetitive tasks and learn, adapt, and make decisions. According to Deloitte, 25% of enterprises using GenAI are forecasted to deploy AI Agents by 2025, growing to 50% by 2027. This trend is driven by the potential for IA to boost efficiency, reduce operational costs, and improve customer experiences.
Intelligent automation in finance is not just a technological upgrade but a strategic necessity for banks and financial institutions aiming to stay competitive.
Let’s start by defining the fundamental elements of IA and discussing how the banking and financial services sectors are changing as a result of its integration.
What is Intelligent Automation in Finance and Banking?
Intelligent automation incorporates various technologies in order to replicate human intelligence in task execution. RPA is primarily focused on automating repetitive tasks, but when combined with AI technologies, it can perform more complex tasks such as sentiment analysis, fraud detection, and predictive analytics.
An excellent example is Capital One’s Eno, an assistant who handles thousands of inquiries while learning from client interactions to offer personalized recommendations.
Rifa AI improves operational efficiency by integrating AI-driven tools that not only speed up debt recovery but also ensure compliance while saving both money and time. If you're automating billing and payment reminders, expediting dispute resolution, or improving customer service interactions, Rifa AI can help you optimize workflows and increase 40% recovery rates.
By using these technologies, banks often achieve more personalized customer experiences and faster financial analytics. After discussing how IA can change business outcomes, let’s examine how these technologies are applied in particular.
Applications and Benefits of Intelligent Automation in Finance
With its many uses and advantages, intelligent automation in finance can have an impact in the following ways:
AI-Led Chatbots to Improve Customer Service
AI-powered chatbots can provide personalized customer support. These bots use NLP to answer consumer questions, assist with financial products, and offer real-time support.
For example, HDFC Bank's Eva chatbot analyzes a customer’s past interactions, like checking credit card rewards or inquiring about loans. If a customer regularly asks about travel-related benefits, Eva might suggest a credit card with better travel perks or a loan with a lower interest rate. This upselling feels seamless and personalized, offering the right service at the right time, based on the customer’s behavior.
Streamlining Back-Office Operations
Automating manual and repetitive back-office processes like procurement, accounts payable, and loan origination saves time and reduces error. RPA tools are commonly used for data extraction, document verification, and compliance checks.
For example, the combination of RPA and Optical Character Recognition (OCR) technology accelerates loan application processing by automatically extracting and verifying data from submitted documents such as tax forms, identification documents, and income proofs. Typically, the OCR system scans and digitizes the documents uploaded by customers, while RPA workflows cross-check this digitized data against internal databases. If discrepancies are identified, such as mismatched income information, the RPA system promptly triggers an alert for further investigation.
Operational Efficiency and Customer Experience
Repetitive tasks can be automated by integrating RPA with AI. While RPA manages structured processes, AI elevates them with features like ML and NLP, enabling the processing of unstructured data such as fraud detection or customer inquiries. Predictive models enable proactive engagement, such as offering personalized financial advice or detecting potential fraud risks early.
For instance, JP Morgan has substantially reduced processing time and operational expenses by using AI to analyze contracts. The technology automatically reviews and scans contracts, extracting relevant details such as payment terms, renewal dates, and highlighting key clauses. By using AI to quickly process large volumes of contracts, JP Morgan has significantly reduced the time spent on manual reviews and minimized human error. The AI system also flags any discrepancies or potential risks, which are then escalated for further examination by human specialists.
Adopting IA helps you stay ahead of the competition by improving customer engagement and streamlining internal processes, which ensures greater dependability and efficiency.
Rifa AI is transforming the process of debt collection by using AI technology to processes and reduce operational costs. By incorporating AI-powered negotiation tools and automating key functions, Rifa AI has proven to improve collection efficiency, shorten recovery cycles, and improve overall payment conversion rates.
Reduce Operational Costs by Up to 70%: Streamline processes to enhance efficiency.
99% Accuracy: AI systems ensure precision in every interaction, minimizing errors.
Quick Deployment: Implement and go live in days without the need for developer or API support.
Agent Time Savings: Automate repetitive tasks, allowing your team to focus on more strategic projects.
2x Improved Payment Conversions: Boost customer engagement and improve payment success rates.
40% Faster Debt Recovery: Accelerate collection cycles and increase cash flow with AI-driven negotiation tools.
Rifa AI runs continuously, ensuring regulatory compliance while seamlessly integrating with your current systems. This transforms your collections and financial operations into more streamlined, customer-centric, and profitable processes.
As we move from technological integration to real-world use cases, it’s critical to acknowledge that implementing IA can present difficulties.
Intelligent Automation Uses
AI, ML, and RPA are among the technologies transforming core banking and financial services operations through intelligent automation in finance. Here are three important use cases:
Automating Regulatory and Compliance Reporting
Manual compliance procedures can be costly, time-consuming, and error-prone. By automating risk assessments, transaction monitoring, and report generation, IA streamlines these processes. Rifa AI fits perfectly into the automation of regulatory and compliance reporting by automating tasks such as transaction monitoring, data extraction, and report generation, helping you maintain up-to-date compliance without the manual effort.
It also simplifies real-time compliance monitoring, ensuring that ever-changing regulations are followed while minimizing human error. By improving accuracy and reducing costs, this automation allows institutions to focus on strategic initiatives and meet regulatory requirements.
Executive Dashboards for Management Reporting
Real-time executive dashboards powered by IA combine data from multiple sources and visualize it to enable prompt decision-making. These dashboards allow executives to monitor operational risks, market trends, and performance metrics. This immediate access to insights optimizes organizational strategies.
During market volatility, a European bank improved the efficiency of C-suite executives' decision-making by using IA to build dashboards that integrated real-time financial metrics.
Improving Data Structuring for Mergers and Acquisitions (M&A)
Analyzing large datasets to evaluate risks and synergy opportunities is a common step in M&A activities. IA organizes and processes unstructured data identifies trends and provides valuable insights. It supports risk assessments, predictive analytics, and integration planning, as well as valuation and due diligence.
A multinational bank used AI-driven automation to analyze operational data, regulatory requirements, and financial records during a recent M&A. This approach provided deeper insights into potential synergies and reduced analysis time by 40%.
By implementing IA, you can boost productivity, reduce costs, and maintain a competitive edge in the financial market.
By using AI-driven negotiation tools to speed up debt recovery and reduce operating expenses by up to 70%, Rifa AI integrates into your current systems. Through the automation of functions such as data extraction, communication management, and payment tracking, Rifa AI helps you maintain compliance. Find out how Rifa AI can optimize your workflows and deliver immediate, cost-effective outcomes.
Addressing key issues like choosing the best technology partners and preparing your staff for upcoming changes will lead to better data management and operational process optimization. Let’s go through IA’s considerations and challenges.
Challenges and Considerations of Intelligent Automation in Finance
Although implementing intelligent automation in finance presents many opportunities, certain challenges should be carefully considered. Key elements of IT integration and cultural change include:
Selection of Vendors and Integration
In finance, IA is often difficult and resource-intensive to integrate with legacy IT systems. Modern automation tools, such as RPA and AI-driven platforms, may need to be supported by the legacy systems that financial institutions typically rely on. To address this:
Work Together Early with IT: A strong partnership with IT teams ensures seamless system lifecycle design and prioritization. To prevent implementation bottlenecks, align automation objectives with IT's overarching strategic roadmap.
Vendor Compatibility: Select solutions that lessen disruptions by enhancing current infrastructure. For example, banks like Barclays have used RPA to streamline back-office operations, resulting in cost savings and increased efficiency without having to redesign their systems completely.
Data Security and Quality: Automation tools require reliable, consistent data. Maintaining strong cybersecurity and data governance is essential to mitigate risks as automation scales. Rifa AI smmothens repetitive tasks, such as communication, reminders, and payment tracking. This automation ensures data accuracy while optimizing efficiency and reducing the risk of human error..
Employee Concerns
The introduction of IA in finance can bring about changes in workplace culture and concerns about job security. Employees may need help to transition into new roles that require more technical skills or fear of being replaced. To overcome these challenges:
Open Communication: Ensure that staff members understand the goals and benefits of automation. Rather than presenting automation as a job replacement, frame it as a tool for reducing repetitive tasks. For instance, organizations like the Australia and New Zealand Banking Group have used automation to free up staff for higher-value tasks.
Invest in Reskilling: Offer reskilling programs to help employees work effectively with automation tools. Promoting a positive attitude through redeployment into strategic roles that emphasize human judgment and creativity is important.
Leadership and Change Champions: To address employee concerns and support automation efforts, engage leaders and early adopters as "change champions." These advocates can help build trust in the transformation process and demonstrate the benefits of intelligent automation in finance.
Both IT integration and cultural adaptation require a phased approach to ensure the technology supports business objectives and gains employee buy-in. By learning from successful case studies and continuously improving procedures, your institution can steer these challenges while optimizing the benefits of automation.
Let’s get into optimizing resources and data integrity, which are the next logical steps in implementing intelligent automation in finance.
Data and Resource Optimization by Intelligent Automation
IA transforms data handling and resource allocation in finance, which uses technologies such as RPA, machine learning, and AI-powered analytics. By applying tools for data integration and validation, you can ensure the reliability of your datasets. This is necessary for compliance, and fraud detection.
Forecasts for the Intelligent Document Processing (IDP) Sector's Growth: The IDP sector, which promotes automated unstructured data extraction, classification, and analysis, is anticipated to grow rapidly. The increasing need for real-time processing in banking tasks such as regulatory reporting, loan approvals, and customer onboarding drives this trend.
Role of ML and NLP: Specifically, the market for IDP is projected to expand significantly due to advances in ML and NLP. These technologies help financial firms manage vast volumes of documents effectively, reduce compliance risks, and increase customer satisfaction.
Rifa AI uses advanced technologies to efficiently manage and process vast amounts of data and documents. By automating the document data extraction process, Rifa AI reduces the likelihood of human error, ensuring high levels of accuracy and compliance. This capability allows businesses to smoothen operations, mitigate compliance risks, and improve operational efficiency.
The ultimate objective of intelligent automation is to increase productivity and reduce operating expenses. Let’s discuss its impact on employees and cost-saving efficiency.
Impact on Employees and Cost Efficiency
Banks can use IA technology to cut expenses, simplify operations, and free up staff members from tedious work, allowing them to take on more strategic responsibilities.
Cutting Back on Working Hours and Strategic Focus: Intelligent automation in finance reduces the amount of manual labor needed for tasks like data entry, document verification, and compliance checks by automating repetitive processes. For example, Standard Chartered’s Trade AI Engine significantly reduces the need for you in routine tasks by using ML to analyze complex trade documents. Employee productivity increases as a result, with more time to concentrate on critical, thinking-intensive tasks like strategy development and customer engagement.
Cost Savings as a Result of Improved Workflows: Intelligent automation reduces costs by replacing inaccurate manual procedures with accurate and reliable automated workflows. This not only improves accuracy but also lowers operating expenses. Compliance and reduced operational risks benefit financial institutions. For example, Banco Santander’s Kairos AI tool promotes reducing the costs associated with risk mismanagement.
The successful adoption of IA by banks improves their operational resilience and positions them as leaders in the industry.
Conclusion
Intelligent automation (IA) in finance is improving customer experiences and operational efficiency. By combining technologies like robotic process automation (RPA), artificial intelligence (AI), and machine learning (ML).
By automating repetitive tasks, IA empowers employees to focus on higher-value activities such as strategic planning and customer engagement, promoting a culture of innovation. Leading banks have used automation platforms to improve data analytics, integrate legacy systems, and roll out new services quickly. Bank operations innovation and competition are all changed by the strategic implementation of intelligent automation in finance.
Rifa AI is in charge of the ongoing revolution of intelligent automation, transforming business collections. In addition to streamlining debt recovery, Rifa AI's AI-driven solutions reduce operating expenses, improve payment conversions, and ensure seamless integration with existing systems.
Jan 2, 2025
Jan 2, 2025
Jan 2, 2025