AI in Payments: Monitoring and Uses
AI in Payments: Monitoring and Uses
AI in Payments: Monitoring and Uses
AI in Payments: Monitoring and Uses
Anant Sharma
Anant Sharma
Anant Sharma
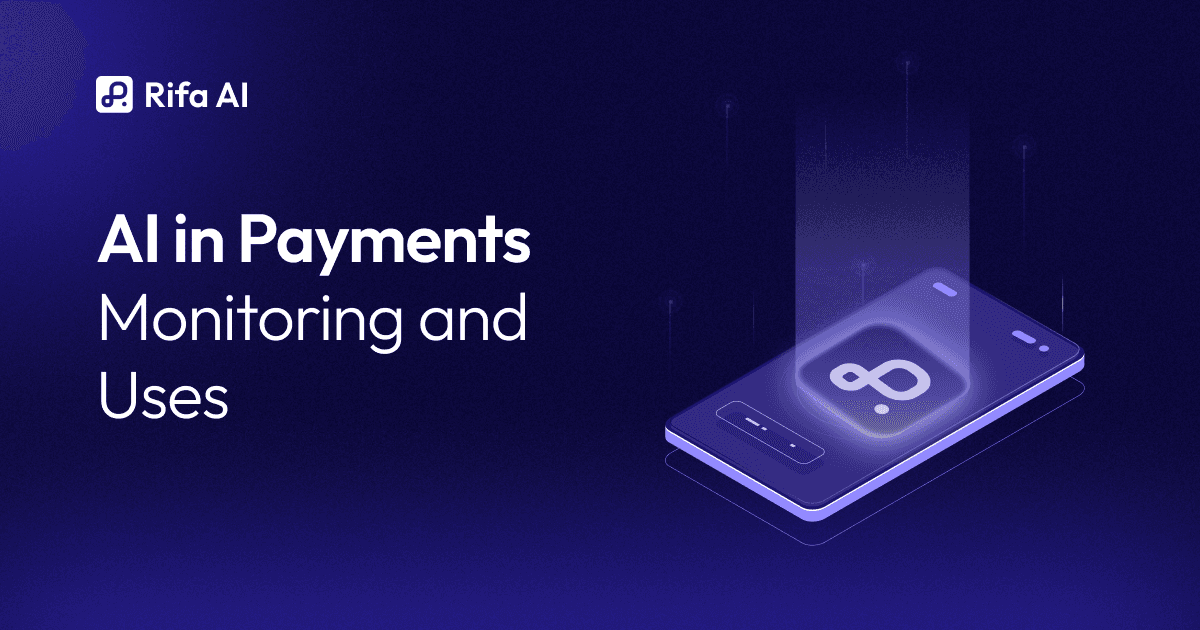
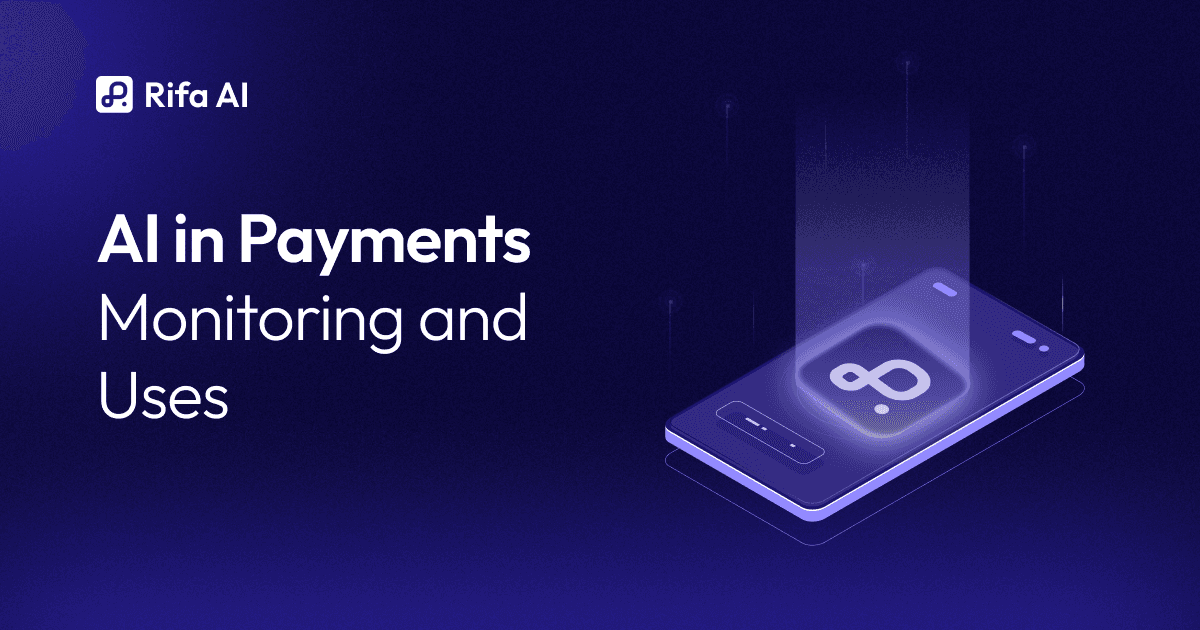
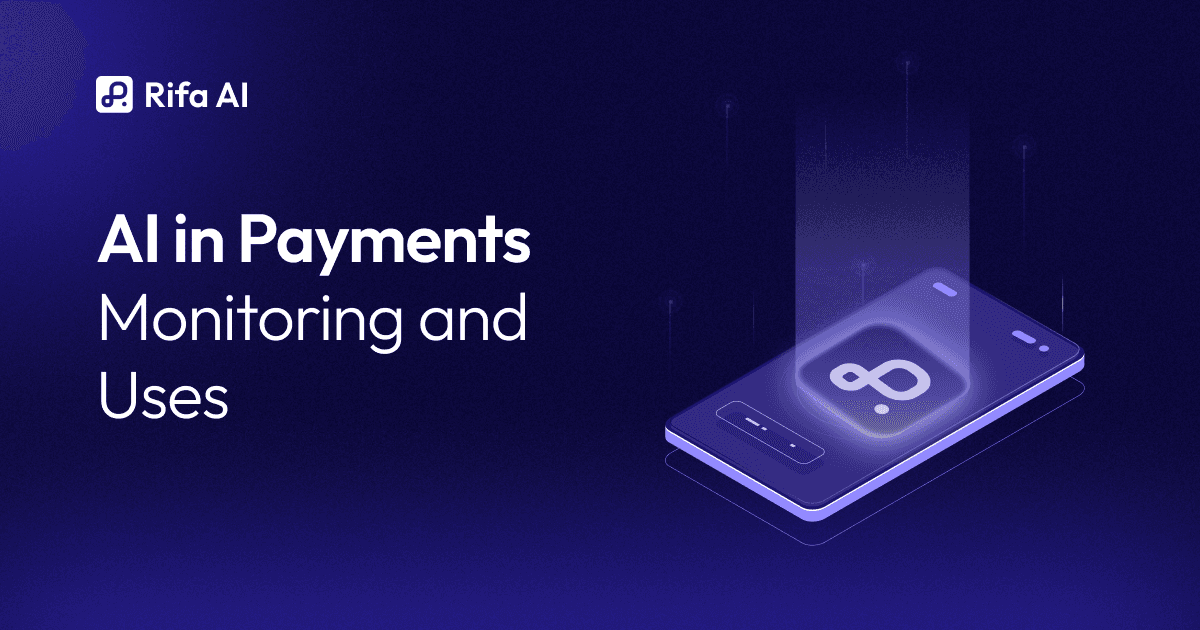
How can companies make sure AI systems monitoring millions of transactions don’t make mistakes or get tampered with? AI is changing how payments are handled, making them faster, safer, and more user-friendly.
With the rise of digital payments worldwide, AI plays a vital role in keeping up with the growing number of transactions.
AI systems can watch millions of transactions at once and flag anything unusual, helping to catch and prevent fraud quickly. For instance, according to MSys Technologies, 89% of adults in the U.S. use digital payments, and nearly two-thirds of adults globally are doing the same.
To handle this massive volume, businesses are using AI tools like Erica, Bank of America’s AI assistant, which helps customers with transactions and advice. VisaNet+AI, another example, uses AI to improve fraud detection and approve transactions more accurately.
This approach of AI for payment monitoring is also paving the way for newer payment methods, such as tap-and-pay systems and financial services embedded directly into apps, making payments easier and more efficient for everyone.
Let’s examine how AI is changing payment monitoring and transforming consumer and business interactions with payment systems.
Understanding AI for Payment Monitoring
To improve efficiency, security, and user experience, AI is being incorporated into payment systems to address major issues with traditional methods and introduce advanced monitoring capabilities.
Drawbacks of Traditional Payment Systems and the Need for AI Integration
Conventional payment methods often face inefficiencies such as slow transaction speeds, vulnerability to fraud, and error-prone manual processes. For example:
Fraudulent Transactions: Rule-based fraud detection, a core component of legacy systems, struggles to keep up with evolving threats. Fraudsters frequently exploit the static nature of these systems with increasingly sophisticated methods.
Scalability Issues: Traditional systems may lack the capacity to handle high transaction volumes, leading to delays and inefficiencies.
Data Analysis Limitations: The limited ability of legacy systems to process and analyze large datasets in real-time reduces their effectiveness in identifying patterns or anomalies.
Rifa AI addresses these challenges by integrating predictive analytics into financial systems, enabling robust recognition of data patterns. Through real-time data analysis, task automation, and predictive decision-making, AI solves these inefficiencies. For instance, AI-driven solutions can dynamically adapt to changing transaction patterns, improving fraud detection and operational efficiency.
Improved Monitoring Capabilities Through AI
AI betters monitoring in payment systems by providing powerful tools for detecting threats, ensuring compliance, and optimizing processes.
Fraud Detection: AI-driven systems use ML algorithms to identify irregularities in transaction patterns. By distinguishing between genuine and fraudulent transactions, these systems reduce false positives and negatives.
For example, many financial institutions use AI to detect unusual spending trends in real-time, preventing fraud before it occurs.
Credit Risk Analysis: AI evaluates a variety of data points, such as transaction history and social behaviors, to assess creditworthiness. This leads to faster and more accurate credit risk assessments, enabling better loan underwriting decisions.
Reconciliation and Automation: AI streamlines payment reconciliation by automatically matching transactions with records and quickly identifying discrepancies. This reduces manual intervention, minimizes errors, and ensures smoother operations.
Improved User Experiences: AI personalizes payment experiences by analyzing user behavior. For instance, AI-powered systems can recommend optimal payment schedules or methods based on a user’s spending habits, boosting customer loyalty and satisfaction.
Integrating AI for payment monitoring transforms monitoring capabilities and addresses traditional inefficiencies. Using AI's predictive and real-time processing abilities can create secure, efficient, and user-friendly payment ecosystems.
However, for successful implementation, challenges like data privacy, regulatory compliance, and algorithmic bias must be carefully managed. AI's capabilities are transforming payment monitoring in numerous ways. Let’s explore the key advantages that make AI indispensable in this field.
Rifa AI offers a powerful solution to the inefficiencies and vulnerabilities of traditional payment systems.
Reduce Operational Costs by Up to 70%: Streamline processes to improve efficiency.
99% Accuracy: AI systems ensure precision in every interaction, minimizing errors.
40% Faster Debt Recovery: Accelerate collection cycles and increase cash flow with AI-driven negotiation tools.
2x Improved Payment Conversions: Boost customer engagement and improve payment success rates.
Agent Time Savings: Automate repetitive tasks, allowing your team to focus on more strategic projects.
Quick Deployment: Implement and go live in days without the need for developer or API support.
Improving data integrity in reconciliation, we have the solutions you need to achieve faster, more accurate results. Contact Rifa AI today and take the first step towards smarter, more secure financial management.
Benefits of AI for Payment Monitoring
Making decisions in real-time, AI demonstrates its potential through its advantages in payment monitoring and real-world applications.
Improved Fraud Detection and Prevention: Higher Security
AI helps safeguard payment systems by instantly detecting and preventing fraudulent activity. It analyzes patterns, highlights irregularities, and adapts to evolving fraud strategies using machine learning algorithms.
For example, imagine a credit card being used in two different countries within minutes. AI for payment monitoring systems can detect this unusual activity, halt the transaction, and promptly notify the cardholder.
Effective AI-based fraud prevention tools, such as Mastercard's Decision Intelligence and Visa's Advanced Authorization system, save billions of dollars annually.
By adopting AI, you can reduce reliance on traditional rule-based systems, which often fail to detect sophisticated fraud schemes. AI provides proactive and dynamic fraud detection by continuously learning from new data.
Better Efficiency: Lower Manual Workloads and Faster Processing
AI for payment monitoring automates repetitive tasks, reducing errors and speeding up transaction approvals. This eliminates the need for manual reviews and outdated procedures, leading to more efficient operations.
For instance, Rifa AI automates time-consuming tasks like fraud reviews, compliance checks, and reconciliation, streamlining payment processes. As a result, transactions are approved faster, accuracy is boosted, and human workloads are reduced. Additionally, AI minimizes false declines by better understanding transaction contexts, offering customers a smoother payment experience.
Real-Time Decision-Making: Instant Insights from Transaction Analysis
AI analyzes transaction data in real-time to provide actionable insights. This allows for instant decision-making, such as dynamically adjusting transaction limits, offering personalized customer experiences, or approving or flagging payments.
For example, e-commerce platforms like Amazon use AI to monitor customer purchasing patterns. If you've ever been offered personalized payment options at checkout, such as installment plans or dynamic pricing, AI is analyzing your behavior and preferences in real-time to tailor those options.
By implementing AI for payment monitoring, you can improve the security, efficiency, and intelligence of your transaction processes while delivering a better experience for your customers.
Rifa AI offers state-of-the-art solutions to automate tedious tasks, detect fraud with 99% accuracy, and deliver immediate insights for faster approvals and personalized customer experiences. Simplify your business processes and build consumer confidence. Discover how Rifa AI can elevate your business toward smarter, safer, and more efficient financial management.
The techniques AI employs to monitor payments are what make it effective. Here’s a closer look at how these cutting-edge tools improve efficiency and security.
AI for Payment Monitoring Techniques
AI for payment monitoring has improved how businesses manage transactions, identify irregularities, and resolve issues in the payments sector. Here are some practical use cases of AI in real-time monitoring:
Real-Time Alerts and Monitoring
AI enables continuous monitoring of payment systems, detecting anomalies such as failed payments, unusual transaction volumes, or unexpected delays.
With real-time analytics, performance issues can be proactively isolated, potential revenue loss prevented, and risks like fraud mitigated.
For instance, AI-based monitoring solutions from Anodot can detect irregularities in millions of transactions and immediately send alerts to prevent disruptions.
This functionality ensures payment processing and protects against operational breakdowns for both businesses and their customers.
ML for Pattern Recognition and Anomaly Detection
ML improves fraud detection by identifying patterns in transaction data. For example, Rifa AI uses advanced ML algorithms to analyze factors such as transaction time, location, and frequency, improving fraud prevention. This approach not only reduces false positives and negatives but also helps detect unusual behaviors.
Autonomous Issue Resolution
AI can identify problems and resolve them independently. Cognitive engines like IPSoft’s Amelia interact with customers to address payment issues without human intervention, learning from past interactions to improve accuracy over time.
Integrating AI for payment monitoring is essential for maintaining competitiveness, boosting customer satisfaction, and ensuring operational efficiency. By using advanced monitoring and ML algorithms, you can optimize payment processes, improve security, and maximize revenue streams.
AI is advancing the way payment systems operate, going beyond just monitoring. Let’s explore its primary applications.
Key Uses of AI for Payment Monitoring
The use of AI for payment monitoring is bettering user experience, efficiency, and security. Here are the main applications of AI in payment systems:
Identifying and Preventing Fraud
AI excels at detecting and preventing fraud by analyzing data in real-time and identifying patterns. Machine learning algorithms analyze large datasets to detect irregularities that indicate fraudulent activities, such as unauthorized transactions or login attempts.
For instance, supervised models are trained using historical data to predict and prevent fraud, while unsupervised learning models identify deviations from normal consumer behavior.
Similar to systems used by PayPal, which focus on minimizing false positives and swiftly identifying genuine threats, Rifa AI continuously learns from historical and real-time data to stay ahead of evolving fraud tactics.
Predictive Analytics
AI employs predictive analytics to forecast transaction volumes and assess risks. This capability enables financial institutions to reduce risk exposure and optimize their resources.
By analyzing transaction history patterns, AI can reveal peak transaction times and potential system bottlenecks.
Predictive models are also used in risk management and credit scoring, allowing businesses to make well-informed decisions regarding lending and fraud prevention. For example, banks use AI to predict fraud patterns and provide proactive protection against emerging threats.
Automation for Customer Service
AI-powered virtual assistants and chatbots improve customer service by addressing queries related to transactions, account information, and payments. These tools offer round-the-clock support, reducing the workload on human support teams and improving response times.
Advanced AI systems can also handle complex queries by analyzing past interactions and providing personalized solutions.
In payment processing, AI-powered interactive voice response (IVR) systems ensure seamless user experiences and escalate complex cases to human agents when needed.
By implementing these AI applications, payment systems can deliver secure, efficient, and customer-centric solutions, driving innovation in the financial sector.
Rifa AI detects real-time fraud detection and prevention with 99% accuracy, resource optimization through transaction volume prediction, and improved customer service through AI-driven chatbots and virtual assistants. Get Rifa AI to help your company stay ahead of the curve and provide safe and easy payment solutions.
As AI continues to evolve, new tools are emerging to improve the payment experience. Let’s get into these innovative advancements.
Advanced AI Tools for Payments
NLP, generative AI, and OCR are just a few of the tools being used to integrate AI in payments, leading to major improvements in security, operational efficiency, and customer interactions.
Here are some examples that demonstrate the transformative potential of advanced AI tools like NLP, generative AI, and OCR in payments.
NLP for Improved Communication
NLP enables chatbots and virtual assistants to interact with customers more intuitively during payment processes. Using simple text or voice commands, users can conduct transactions, check account balances, or resolve payment issues with conversational banking tools powered by NLP.
A noteworthy example is Microsoft’s integration of language models into programs like Excel, allowing users to query financial data and easily derive actionable insights conversationally.
To drastically reduce wait times and improve customer satisfaction, banks and payment platforms use NLP to provide real-time responses and insights.
Customized Payment Experiences with Generative AI
Generative AI enables highly customized payment systems. By analyzing user data, it can offer personalized rewards, dynamic pricing, or product recommendations.
For instance, when a customer interacts with a merchant's chatbot, AI can streamline the shopping and payment process by suggesting products based on the customer’s preferences and past purchases.
Generative AI also helps prevent fraud by detecting suspicious transactions before they are processed and predicting fraudulent patterns based on large datasets.
Rifa AI, for example, can quickly digitize physical documents such as invoices, receipts, and KYC forms, making back-office operations like customer onboarding and payment reconciliation more efficient.
OCR (Optical Character Recognition) for Automating Documents
OCR eliminates errors and delays associated with manual data entry by extracting and digitizing information from physical documents like identification cards, invoices, and receipts. For instance, financial institutions use OCR to expedite Know Your Customer (KYC) procedures, speeding up customer onboarding. OCR also betters payment reconciliation by automatically matching invoices and payment records.
Additionally, OCR is useful in applications like automated expense tracking. Employees can speed up the reimbursement process by scanning receipts, and AI systems can automatically generate expense reports by extracting relevant data.
These technologies allow payment providers to stay competitive in a rapidly changing market while improving the user experience. However, challenges like data privacy, legal compliance, and the need for responsible AI for payment monitoring still hinder widespread adoption.
The true impact of AI is best demonstrated through its successful applications. Here are some notable ways leading businesses are using AI in payments.
Successful Use Cases in AI for Payment Monitoring
AI for payment monitoring is improving customer experiences, increasing fraud detection, and optimizing operations. Let’s examine some effective AI use cases from widely used companies like Stripe, Visa, Mastercard, and PayPal.
Integration of AI by PayPal and Mastercard
To minimize consumer disruption, MasterCard’s Safety Net platform employs AI to detect and stop fraud attempts before they reach issuing banks. To further improve their fraud detection algorithms, they also use generative AI to simulate artificial transaction data.
Additionally, by enabling users to pay using hand gestures or facial recognition, Mastercard’s Biometric Checkout Program streamlines payments while improving security and convenience.
PayPal uses virtual assistants powered by artificial intelligence to handle more than 30 million customer inquiries every year. This method improves customer service efficiency and satisfaction by lowering call center volumes and providing round-the-clock assistance for questions regarding account balances, transaction disputes, and other topics.
Visa’s Use of AI for Risk Management and Fraud Detection
Visa uses AI to examine transaction data in real-time, spot irregularities, and reduce the risk of fraud. By identifying patterns in customer behavior, their AI models allow them to prevent fraudulent activity before it starts.
To illustrate AI’s ability to track and respond to behavioral insights, Visa’s machine learning systems, for example, discovered unusual increases in spending patterns, such as a rise in gaming and streaming subscriptions during specific times.
AI-Powered Credit Monitoring and Decisioning on Stripe
Stripe uses machine learning to assess merchant risk profiles. It analyzes over 1,500 variables for real-time credit decisions in 200+ countries. By approving 20% more applications without raising default rates, this system increases underwriting efficiency.
Through the processing of various data points, including credit history and financial behavior, Stripe makes it possible to make better credit decisions and increase accessibility for users with short credit histories.
Personalized underwriting by Stripe and fraud detection by Mastercard and Visa are just two examples of how AI is opening the door to a safer and easier payment ecosystem.
Rifa AI can help you double payment conversions and accelerate debt recovery by 40%, with our advanced fraud detection system ensuring 99% accuracy in every transaction and task automation, freeing up agent time. Get Rifa AI and let your team focus on more important projects.
Despite its advantages, the integration of AI for payment monitoring comes with its own set of challenges. Let’s examine the key issues and potential solutions.
Challenges and Considerations of AI for Payment Monitoring
The application of AI for payment monitoring introduces capabilities but also comes Although the use of AI in payments offers revolutionary potential, there are also important issues and concerns to take into account.
Here’s how to tackle these problems successfully:
Information Security and Privacy
Payment systems are vulnerable to breaches and misuse due to AI's reliance on enormous volumes of sensitive data. You must prioritize strong encryption, use zero-trust architectures, and ensure that data is stored and transmitted securely in order to reduce risks.
Data breaches can be avoided by implementing AI tools that use real-time anomaly monitoring. For example, Mastercard reduces fraud losses while protecting customer data by using AI to proactively detect fraud by analyzing billions of transactions every day.
The Bias of Algorithms
Biases may be unintentionally reinforced or worsened by AI algorithms, especially when it comes to fraud detection or credit risk assessment. Regular audits of AI models, transparency in decision-making procedures, and training datasets that reduce inherent biases are all necessary to ensure fairness.
For instance, using explainable AI (XAI) makes it possible to track the reasoning behind decisions, guaranteeing adherence to anti-discrimination regulations.
To provide transparent decision-making processes, Rifa AI integrates XAI techniques. By doing this, companies can verify and track the reasoning behind automated decisions, ensuring adherence to anti-discrimination regulations and promoting user confidence.
Compliance with Regulations
Because the legal framework governing AI in payments is constantly changing, businesses must maintain compliance with both domestic and international laws. Maintaining data privacy in accordance with GDPR and ensuring fairness in automated decision-making to comply with regulations.
Building trust with regulators can be achieved through cooperation, such as exchanging model validation techniques. For example, Microsoft's use of generative AI in legal teams shows how using AI can simplify regulatory compliance.
You can maximize AI's potential in payments while lowering related risks by taking these factors into account.
Conclusion
AI is changing how payment systems work by making them faster, safer, and more customer-friendly. It uses advanced technology like ML to study large amounts of data and spot unusual activities.
AI for payment monitoring helps businesses personalize their services by offering recommendations and deals tailored to each customer’s preferences. This makes customers feel valued and improves their overall experience, encouraging loyalty.
Looking ahead, AI will work with technologies like biometrics and blockchain. Blockchain can make international payments more secure and transparent, while biometric systems (like facial recognition or fingerprint scanning) ensure safe and easy payment methods.
Innovations, such as Central Bank Digital Currencies (CBDCs) and AI-powered digital wallets, will make payments more inclusive and efficient for everyone.
However, challenges like protecting data privacy, avoiding errors in AI systems, and following legal rules remain. To get the most out of AI, businesses need to focus on ethical practices and strengthen their cybersecurity measures.
Rifa AI guarantees safe, and effective payment solutions, from fraud detection to customized customer experiences. With Rifa AI, you can improve customer experiences with tailored services, detect fraud with unparalleled precision, and streamline payment processes for optimal efficiency.
Schedule a demo toward smarter, more reliable, and customer-centric systems with Rifa AI today. Your gateway to the future of secure, efficient, and customer-focused payment solutions is Rifa AI.
How can companies make sure AI systems monitoring millions of transactions don’t make mistakes or get tampered with? AI is changing how payments are handled, making them faster, safer, and more user-friendly.
With the rise of digital payments worldwide, AI plays a vital role in keeping up with the growing number of transactions.
AI systems can watch millions of transactions at once and flag anything unusual, helping to catch and prevent fraud quickly. For instance, according to MSys Technologies, 89% of adults in the U.S. use digital payments, and nearly two-thirds of adults globally are doing the same.
To handle this massive volume, businesses are using AI tools like Erica, Bank of America’s AI assistant, which helps customers with transactions and advice. VisaNet+AI, another example, uses AI to improve fraud detection and approve transactions more accurately.
This approach of AI for payment monitoring is also paving the way for newer payment methods, such as tap-and-pay systems and financial services embedded directly into apps, making payments easier and more efficient for everyone.
Let’s examine how AI is changing payment monitoring and transforming consumer and business interactions with payment systems.
Understanding AI for Payment Monitoring
To improve efficiency, security, and user experience, AI is being incorporated into payment systems to address major issues with traditional methods and introduce advanced monitoring capabilities.
Drawbacks of Traditional Payment Systems and the Need for AI Integration
Conventional payment methods often face inefficiencies such as slow transaction speeds, vulnerability to fraud, and error-prone manual processes. For example:
Fraudulent Transactions: Rule-based fraud detection, a core component of legacy systems, struggles to keep up with evolving threats. Fraudsters frequently exploit the static nature of these systems with increasingly sophisticated methods.
Scalability Issues: Traditional systems may lack the capacity to handle high transaction volumes, leading to delays and inefficiencies.
Data Analysis Limitations: The limited ability of legacy systems to process and analyze large datasets in real-time reduces their effectiveness in identifying patterns or anomalies.
Rifa AI addresses these challenges by integrating predictive analytics into financial systems, enabling robust recognition of data patterns. Through real-time data analysis, task automation, and predictive decision-making, AI solves these inefficiencies. For instance, AI-driven solutions can dynamically adapt to changing transaction patterns, improving fraud detection and operational efficiency.
Improved Monitoring Capabilities Through AI
AI betters monitoring in payment systems by providing powerful tools for detecting threats, ensuring compliance, and optimizing processes.
Fraud Detection: AI-driven systems use ML algorithms to identify irregularities in transaction patterns. By distinguishing between genuine and fraudulent transactions, these systems reduce false positives and negatives.
For example, many financial institutions use AI to detect unusual spending trends in real-time, preventing fraud before it occurs.
Credit Risk Analysis: AI evaluates a variety of data points, such as transaction history and social behaviors, to assess creditworthiness. This leads to faster and more accurate credit risk assessments, enabling better loan underwriting decisions.
Reconciliation and Automation: AI streamlines payment reconciliation by automatically matching transactions with records and quickly identifying discrepancies. This reduces manual intervention, minimizes errors, and ensures smoother operations.
Improved User Experiences: AI personalizes payment experiences by analyzing user behavior. For instance, AI-powered systems can recommend optimal payment schedules or methods based on a user’s spending habits, boosting customer loyalty and satisfaction.
Integrating AI for payment monitoring transforms monitoring capabilities and addresses traditional inefficiencies. Using AI's predictive and real-time processing abilities can create secure, efficient, and user-friendly payment ecosystems.
However, for successful implementation, challenges like data privacy, regulatory compliance, and algorithmic bias must be carefully managed. AI's capabilities are transforming payment monitoring in numerous ways. Let’s explore the key advantages that make AI indispensable in this field.
Rifa AI offers a powerful solution to the inefficiencies and vulnerabilities of traditional payment systems.
Reduce Operational Costs by Up to 70%: Streamline processes to improve efficiency.
99% Accuracy: AI systems ensure precision in every interaction, minimizing errors.
40% Faster Debt Recovery: Accelerate collection cycles and increase cash flow with AI-driven negotiation tools.
2x Improved Payment Conversions: Boost customer engagement and improve payment success rates.
Agent Time Savings: Automate repetitive tasks, allowing your team to focus on more strategic projects.
Quick Deployment: Implement and go live in days without the need for developer or API support.
Improving data integrity in reconciliation, we have the solutions you need to achieve faster, more accurate results. Contact Rifa AI today and take the first step towards smarter, more secure financial management.
Benefits of AI for Payment Monitoring
Making decisions in real-time, AI demonstrates its potential through its advantages in payment monitoring and real-world applications.
Improved Fraud Detection and Prevention: Higher Security
AI helps safeguard payment systems by instantly detecting and preventing fraudulent activity. It analyzes patterns, highlights irregularities, and adapts to evolving fraud strategies using machine learning algorithms.
For example, imagine a credit card being used in two different countries within minutes. AI for payment monitoring systems can detect this unusual activity, halt the transaction, and promptly notify the cardholder.
Effective AI-based fraud prevention tools, such as Mastercard's Decision Intelligence and Visa's Advanced Authorization system, save billions of dollars annually.
By adopting AI, you can reduce reliance on traditional rule-based systems, which often fail to detect sophisticated fraud schemes. AI provides proactive and dynamic fraud detection by continuously learning from new data.
Better Efficiency: Lower Manual Workloads and Faster Processing
AI for payment monitoring automates repetitive tasks, reducing errors and speeding up transaction approvals. This eliminates the need for manual reviews and outdated procedures, leading to more efficient operations.
For instance, Rifa AI automates time-consuming tasks like fraud reviews, compliance checks, and reconciliation, streamlining payment processes. As a result, transactions are approved faster, accuracy is boosted, and human workloads are reduced. Additionally, AI minimizes false declines by better understanding transaction contexts, offering customers a smoother payment experience.
Real-Time Decision-Making: Instant Insights from Transaction Analysis
AI analyzes transaction data in real-time to provide actionable insights. This allows for instant decision-making, such as dynamically adjusting transaction limits, offering personalized customer experiences, or approving or flagging payments.
For example, e-commerce platforms like Amazon use AI to monitor customer purchasing patterns. If you've ever been offered personalized payment options at checkout, such as installment plans or dynamic pricing, AI is analyzing your behavior and preferences in real-time to tailor those options.
By implementing AI for payment monitoring, you can improve the security, efficiency, and intelligence of your transaction processes while delivering a better experience for your customers.
Rifa AI offers state-of-the-art solutions to automate tedious tasks, detect fraud with 99% accuracy, and deliver immediate insights for faster approvals and personalized customer experiences. Simplify your business processes and build consumer confidence. Discover how Rifa AI can elevate your business toward smarter, safer, and more efficient financial management.
The techniques AI employs to monitor payments are what make it effective. Here’s a closer look at how these cutting-edge tools improve efficiency and security.
AI for Payment Monitoring Techniques
AI for payment monitoring has improved how businesses manage transactions, identify irregularities, and resolve issues in the payments sector. Here are some practical use cases of AI in real-time monitoring:
Real-Time Alerts and Monitoring
AI enables continuous monitoring of payment systems, detecting anomalies such as failed payments, unusual transaction volumes, or unexpected delays.
With real-time analytics, performance issues can be proactively isolated, potential revenue loss prevented, and risks like fraud mitigated.
For instance, AI-based monitoring solutions from Anodot can detect irregularities in millions of transactions and immediately send alerts to prevent disruptions.
This functionality ensures payment processing and protects against operational breakdowns for both businesses and their customers.
ML for Pattern Recognition and Anomaly Detection
ML improves fraud detection by identifying patterns in transaction data. For example, Rifa AI uses advanced ML algorithms to analyze factors such as transaction time, location, and frequency, improving fraud prevention. This approach not only reduces false positives and negatives but also helps detect unusual behaviors.
Autonomous Issue Resolution
AI can identify problems and resolve them independently. Cognitive engines like IPSoft’s Amelia interact with customers to address payment issues without human intervention, learning from past interactions to improve accuracy over time.
Integrating AI for payment monitoring is essential for maintaining competitiveness, boosting customer satisfaction, and ensuring operational efficiency. By using advanced monitoring and ML algorithms, you can optimize payment processes, improve security, and maximize revenue streams.
AI is advancing the way payment systems operate, going beyond just monitoring. Let’s explore its primary applications.
Key Uses of AI for Payment Monitoring
The use of AI for payment monitoring is bettering user experience, efficiency, and security. Here are the main applications of AI in payment systems:
Identifying and Preventing Fraud
AI excels at detecting and preventing fraud by analyzing data in real-time and identifying patterns. Machine learning algorithms analyze large datasets to detect irregularities that indicate fraudulent activities, such as unauthorized transactions or login attempts.
For instance, supervised models are trained using historical data to predict and prevent fraud, while unsupervised learning models identify deviations from normal consumer behavior.
Similar to systems used by PayPal, which focus on minimizing false positives and swiftly identifying genuine threats, Rifa AI continuously learns from historical and real-time data to stay ahead of evolving fraud tactics.
Predictive Analytics
AI employs predictive analytics to forecast transaction volumes and assess risks. This capability enables financial institutions to reduce risk exposure and optimize their resources.
By analyzing transaction history patterns, AI can reveal peak transaction times and potential system bottlenecks.
Predictive models are also used in risk management and credit scoring, allowing businesses to make well-informed decisions regarding lending and fraud prevention. For example, banks use AI to predict fraud patterns and provide proactive protection against emerging threats.
Automation for Customer Service
AI-powered virtual assistants and chatbots improve customer service by addressing queries related to transactions, account information, and payments. These tools offer round-the-clock support, reducing the workload on human support teams and improving response times.
Advanced AI systems can also handle complex queries by analyzing past interactions and providing personalized solutions.
In payment processing, AI-powered interactive voice response (IVR) systems ensure seamless user experiences and escalate complex cases to human agents when needed.
By implementing these AI applications, payment systems can deliver secure, efficient, and customer-centric solutions, driving innovation in the financial sector.
Rifa AI detects real-time fraud detection and prevention with 99% accuracy, resource optimization through transaction volume prediction, and improved customer service through AI-driven chatbots and virtual assistants. Get Rifa AI to help your company stay ahead of the curve and provide safe and easy payment solutions.
As AI continues to evolve, new tools are emerging to improve the payment experience. Let’s get into these innovative advancements.
Advanced AI Tools for Payments
NLP, generative AI, and OCR are just a few of the tools being used to integrate AI in payments, leading to major improvements in security, operational efficiency, and customer interactions.
Here are some examples that demonstrate the transformative potential of advanced AI tools like NLP, generative AI, and OCR in payments.
NLP for Improved Communication
NLP enables chatbots and virtual assistants to interact with customers more intuitively during payment processes. Using simple text or voice commands, users can conduct transactions, check account balances, or resolve payment issues with conversational banking tools powered by NLP.
A noteworthy example is Microsoft’s integration of language models into programs like Excel, allowing users to query financial data and easily derive actionable insights conversationally.
To drastically reduce wait times and improve customer satisfaction, banks and payment platforms use NLP to provide real-time responses and insights.
Customized Payment Experiences with Generative AI
Generative AI enables highly customized payment systems. By analyzing user data, it can offer personalized rewards, dynamic pricing, or product recommendations.
For instance, when a customer interacts with a merchant's chatbot, AI can streamline the shopping and payment process by suggesting products based on the customer’s preferences and past purchases.
Generative AI also helps prevent fraud by detecting suspicious transactions before they are processed and predicting fraudulent patterns based on large datasets.
Rifa AI, for example, can quickly digitize physical documents such as invoices, receipts, and KYC forms, making back-office operations like customer onboarding and payment reconciliation more efficient.
OCR (Optical Character Recognition) for Automating Documents
OCR eliminates errors and delays associated with manual data entry by extracting and digitizing information from physical documents like identification cards, invoices, and receipts. For instance, financial institutions use OCR to expedite Know Your Customer (KYC) procedures, speeding up customer onboarding. OCR also betters payment reconciliation by automatically matching invoices and payment records.
Additionally, OCR is useful in applications like automated expense tracking. Employees can speed up the reimbursement process by scanning receipts, and AI systems can automatically generate expense reports by extracting relevant data.
These technologies allow payment providers to stay competitive in a rapidly changing market while improving the user experience. However, challenges like data privacy, legal compliance, and the need for responsible AI for payment monitoring still hinder widespread adoption.
The true impact of AI is best demonstrated through its successful applications. Here are some notable ways leading businesses are using AI in payments.
Successful Use Cases in AI for Payment Monitoring
AI for payment monitoring is improving customer experiences, increasing fraud detection, and optimizing operations. Let’s examine some effective AI use cases from widely used companies like Stripe, Visa, Mastercard, and PayPal.
Integration of AI by PayPal and Mastercard
To minimize consumer disruption, MasterCard’s Safety Net platform employs AI to detect and stop fraud attempts before they reach issuing banks. To further improve their fraud detection algorithms, they also use generative AI to simulate artificial transaction data.
Additionally, by enabling users to pay using hand gestures or facial recognition, Mastercard’s Biometric Checkout Program streamlines payments while improving security and convenience.
PayPal uses virtual assistants powered by artificial intelligence to handle more than 30 million customer inquiries every year. This method improves customer service efficiency and satisfaction by lowering call center volumes and providing round-the-clock assistance for questions regarding account balances, transaction disputes, and other topics.
Visa’s Use of AI for Risk Management and Fraud Detection
Visa uses AI to examine transaction data in real-time, spot irregularities, and reduce the risk of fraud. By identifying patterns in customer behavior, their AI models allow them to prevent fraudulent activity before it starts.
To illustrate AI’s ability to track and respond to behavioral insights, Visa’s machine learning systems, for example, discovered unusual increases in spending patterns, such as a rise in gaming and streaming subscriptions during specific times.
AI-Powered Credit Monitoring and Decisioning on Stripe
Stripe uses machine learning to assess merchant risk profiles. It analyzes over 1,500 variables for real-time credit decisions in 200+ countries. By approving 20% more applications without raising default rates, this system increases underwriting efficiency.
Through the processing of various data points, including credit history and financial behavior, Stripe makes it possible to make better credit decisions and increase accessibility for users with short credit histories.
Personalized underwriting by Stripe and fraud detection by Mastercard and Visa are just two examples of how AI is opening the door to a safer and easier payment ecosystem.
Rifa AI can help you double payment conversions and accelerate debt recovery by 40%, with our advanced fraud detection system ensuring 99% accuracy in every transaction and task automation, freeing up agent time. Get Rifa AI and let your team focus on more important projects.
Despite its advantages, the integration of AI for payment monitoring comes with its own set of challenges. Let’s examine the key issues and potential solutions.
Challenges and Considerations of AI for Payment Monitoring
The application of AI for payment monitoring introduces capabilities but also comes Although the use of AI in payments offers revolutionary potential, there are also important issues and concerns to take into account.
Here’s how to tackle these problems successfully:
Information Security and Privacy
Payment systems are vulnerable to breaches and misuse due to AI's reliance on enormous volumes of sensitive data. You must prioritize strong encryption, use zero-trust architectures, and ensure that data is stored and transmitted securely in order to reduce risks.
Data breaches can be avoided by implementing AI tools that use real-time anomaly monitoring. For example, Mastercard reduces fraud losses while protecting customer data by using AI to proactively detect fraud by analyzing billions of transactions every day.
The Bias of Algorithms
Biases may be unintentionally reinforced or worsened by AI algorithms, especially when it comes to fraud detection or credit risk assessment. Regular audits of AI models, transparency in decision-making procedures, and training datasets that reduce inherent biases are all necessary to ensure fairness.
For instance, using explainable AI (XAI) makes it possible to track the reasoning behind decisions, guaranteeing adherence to anti-discrimination regulations.
To provide transparent decision-making processes, Rifa AI integrates XAI techniques. By doing this, companies can verify and track the reasoning behind automated decisions, ensuring adherence to anti-discrimination regulations and promoting user confidence.
Compliance with Regulations
Because the legal framework governing AI in payments is constantly changing, businesses must maintain compliance with both domestic and international laws. Maintaining data privacy in accordance with GDPR and ensuring fairness in automated decision-making to comply with regulations.
Building trust with regulators can be achieved through cooperation, such as exchanging model validation techniques. For example, Microsoft's use of generative AI in legal teams shows how using AI can simplify regulatory compliance.
You can maximize AI's potential in payments while lowering related risks by taking these factors into account.
Conclusion
AI is changing how payment systems work by making them faster, safer, and more customer-friendly. It uses advanced technology like ML to study large amounts of data and spot unusual activities.
AI for payment monitoring helps businesses personalize their services by offering recommendations and deals tailored to each customer’s preferences. This makes customers feel valued and improves their overall experience, encouraging loyalty.
Looking ahead, AI will work with technologies like biometrics and blockchain. Blockchain can make international payments more secure and transparent, while biometric systems (like facial recognition or fingerprint scanning) ensure safe and easy payment methods.
Innovations, such as Central Bank Digital Currencies (CBDCs) and AI-powered digital wallets, will make payments more inclusive and efficient for everyone.
However, challenges like protecting data privacy, avoiding errors in AI systems, and following legal rules remain. To get the most out of AI, businesses need to focus on ethical practices and strengthen their cybersecurity measures.
Rifa AI guarantees safe, and effective payment solutions, from fraud detection to customized customer experiences. With Rifa AI, you can improve customer experiences with tailored services, detect fraud with unparalleled precision, and streamline payment processes for optimal efficiency.
Schedule a demo toward smarter, more reliable, and customer-centric systems with Rifa AI today. Your gateway to the future of secure, efficient, and customer-focused payment solutions is Rifa AI.
Jan 2, 2025
Jan 2, 2025
Jan 2, 2025